Looking 3D: Anomaly Detection with 2D-3D Alignment
CVPR 2024
A Novel Task: Conditional AD
Standard Anomaly Detection (AD) frameworks perform pooly without clear defination of ‘normality’, especially when abnormalities are arbitrary and instance-specific. Our paper introduces a novel conditional AD task, along with a new benchmark and an effective solution, that aims to identify and localize anomalies from a photo of an object instance (i.e., the query image), in relation to a reference 3D model.
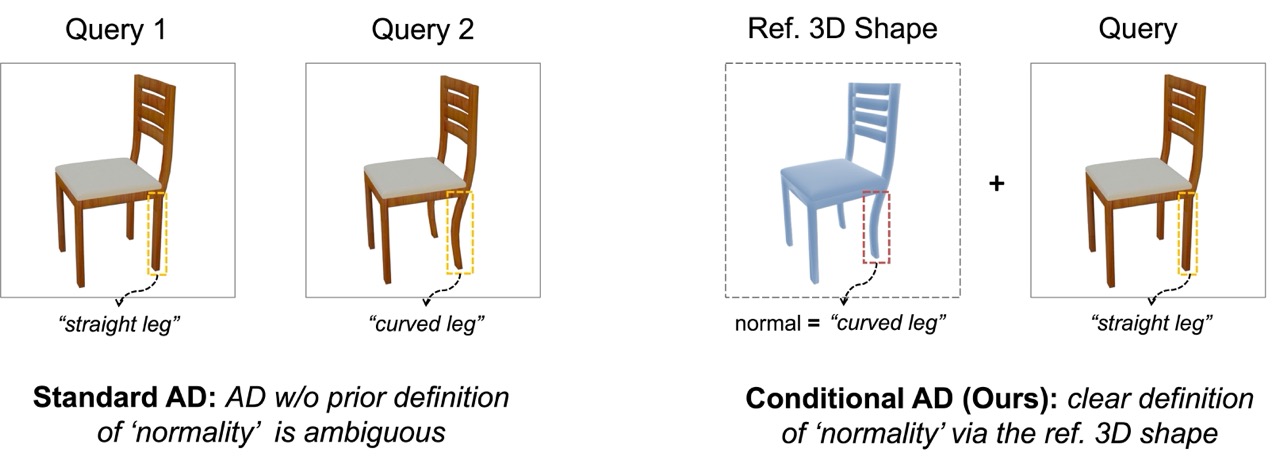
The 3D model provides the reference shape for the regular object instance, and hence a clear definition of regularity for the query image. This setting is motivated by real-world applications in inspection and quality control, where an object instance is manufactured based on a reference 3D model, which can then be used to identify anomalies (i.e., production faults, damages) from a photo of the instance.
BrokenChairs-180K Dataset and Benchmark
As there is no prior large public dataset with paired 3D shapes and images, we introduce BrokenChairs-180K, a new benchmark for the proposed conditional AD task. It consists of around 180K images, with diverse anomalies, geometries, and textures paired with 8,143 reference 3D shapes. Our dataset is derived from PartNet models.

Method: To tackle this task, we have proposed a novel transformer-based approach that explicitly learns the correspondence between the query image and reference 3D shape via feature alignment and leverages a customized attention mechanism for anomaly detection. Our approach has been rigorously evaluated through comprehensive experiments, serving as a benchmark for future research in this domain. The training and testing code of our model is available here.